Constructed with an interpretable module for native bilateral dissimilarity on mammography exams, the deep studying platform AsymMirai might present enhanced readability of the reasoning behind synthetic intelligence (AI) assessments of breast most cancers threat as much as 5 years with comparable AUCs to a beforehand validated black field AI mannequin.
For the retrospective examine, just lately printed in Radiology, researchers in contrast the prognostic functionality of AsymMirai with Mirai, one other deep studying mammography-based modality for assessing breast most cancers threat. Whereas each fashions use commonplace mediolateral indirect and craniocaudal mammography views as inputs, the examine authors famous that Mirai is comprised of a convolutional neural community and a transformer. The transformer-free AsymMirai maintains spatial correspondence between inputted mammography photographs and extracted options and offers one bilateral dissimilarity rating primarily based on common scores for every enter worth, in line with the researchers.
Within the evaluation of 210,067 screening mammograms from 81,824 sufferers, the researchers discovered that AsymMirai supplied comparable AUCs (space underneath the curves) for breast most cancers threat evaluation as Mirai. Particularly, the AsymMirai mannequin, with its emphasis on native bilateral dissimilarity, had one-, three- and five-year AUCs of 79 p.c, 68 p.c and 66 p.c, respectively, compared to 84 p.c, 72 p.c and 71 p.c, respectively, for the Mirai mannequin.
Notice the excessive location consistency with the AsymMirai deep studying evaluation on full-field screening mammography at preliminary imaging in 2014 (left), 17 months later (center) and 21 months after the second examination (proper). The affected person, who was 69 years of age on the 2014 screening was identified with ductal carcinoma in situ (DCIS) in 2020. (Photos courtesy of Radiology.)
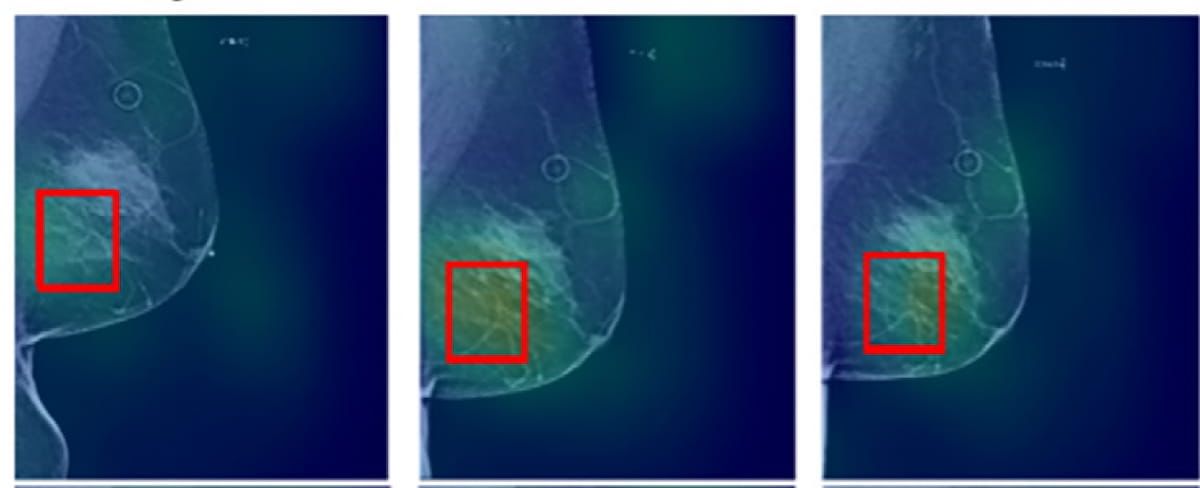
“Utilizing the present Mirai front-end convolutional neural community for characteristic extraction, (AysmMirai) calculates variations within the latent house, offering the placement of the dissimilarity, which is visually intuitive. This rating approximates that of Mirai … with solely a slight discount in 1-5-year threat prediction efficiency,” wrote co-senior writer Cynthia Rudin, Ph.D., a professor of laptop science, electrical and laptop engineering, statistical science and biostatistics and bioinformatics, and director of the Interpretable Machine Studying Lab at Duke College, and colleagues.
Noting the position of location consistency for highlighted tissue and a doable 40 p.c threshold for shift of the prediction window, the researchers famous superior breast most cancers prediction for the AysmMirai mannequin in 383 sufferers who had a window shift of 40 p.c or much less. On this inhabitants, AysmMirai demonstrated a 92 p.c AUC at one yr and an 88 p.c AUC at 5 years.
Three Key Takeaways
- Interpretable AI for breast most cancers threat evaluation. AsymMirai, that includes an interpretable module for native bilateral dissimilarity, might improve the readability of AI assessments in breast most cancers threat prediction. This interpretability aids in understanding the reasoning behind AI-generated predictions, which is essential for scientific acceptance and belief.
- Comparable AUCs with improved reasoning readability. Along with using an interpretable module, AsymMirai demonstrates comparable efficiency to Mirai, a black field AI mannequin, when it comes to space underneath the curves (AUCs) for breast most cancers threat evaluation. This implies that improved interpretability might not considerably compromise predictive accuracy and will supply clinicians a extra clear perception into AI-generated threat assessments.
- Superior predictive efficiency in sure affected person subgroups. AsymMirai displays superior breast most cancers prediction, notably in sufferers with a restricted shift within the prediction window (40% or much less), exhibiting greater AUCs at each one (92 p.c) and 5 years (88 p.c). The examine authors indicated the potential of AsymMirai to detect abnormalities in breast tissue earlier, presumably facilitating extra well timed intervention or threat administration methods. Nevertheless, the efficiency distinction between AsymMirai and Mirai varies throughout demographic teams, with Mirai performing higher for sufferers aged 50 to 70 and African American ladies, highlighting the significance of contemplating demographic components in AI-based threat evaluation fashions.
“We initially anticipated this to be the case as a result of AysmMirai would discover abnormalities within the tissue earlier than the event of the particular lesion,” added Rudin and colleagues. “Whereas this does happen, most sufferers with location consistency of 40% or much less confirmed little change from prior examinations and thus correspond to a really low-risk group … .”
The analysis findings confirmed bigger AUC variations between the AsymMirai and Mirai fashions for sufferers between 50 to 70 years of age (ranging between six to eight p.c greater AUCs for Mirai) and African American ladies (ranging between six to 9 p.c greater for Mirai)
(Editor’s be aware: For associated content material, see “Mammography-Based mostly Deep Studying Mannequin Facilitates Greater Breast Most cancers Detection on Screening MRI,” “Mammography-Based mostly Deep Studying Mannequin Could Assist Detect Precancerous Adjustments in Excessive-Danger Ladies” and “Deep Studying Detection of Mammography Abnormalities: What a New Research Reveals.”)
Past the inherent limitations of a retrospective examine, the examine authors acknowledged that bilateral dissimilarity will not be the only foundation for predictions made with the Mirai platform. Noting that African American sufferers comprised 3.75 p.c of the coaching knowledge set for the Mirai system, the researchers conceded the modality lacks equal efficiency throughout racial teams. The researchers additionally identified, with respect to location consistency on mammography exams, that five-year follow-up knowledge was solely accessible for 10.7 p.c of those that had a 40 p.c or decrease window shift.